Overarching Foci
Longitudinal data subject to irregular observation
Clinic based cohorts are an efficient and low-cost approach to gathering longitudinal data and are used by several research groups at SickKids. Patients are enrolled in the cohort at diagnosis, followed up as medically necessary, and data are gathered through a chart review. However, since patients tend to visit more often when unwell, this can lead to overestimation of the burden of disease unless accounted for appropriately. We work on methods to mitigate this bias. Many of these methods have been incorporated into our R package IrregLong
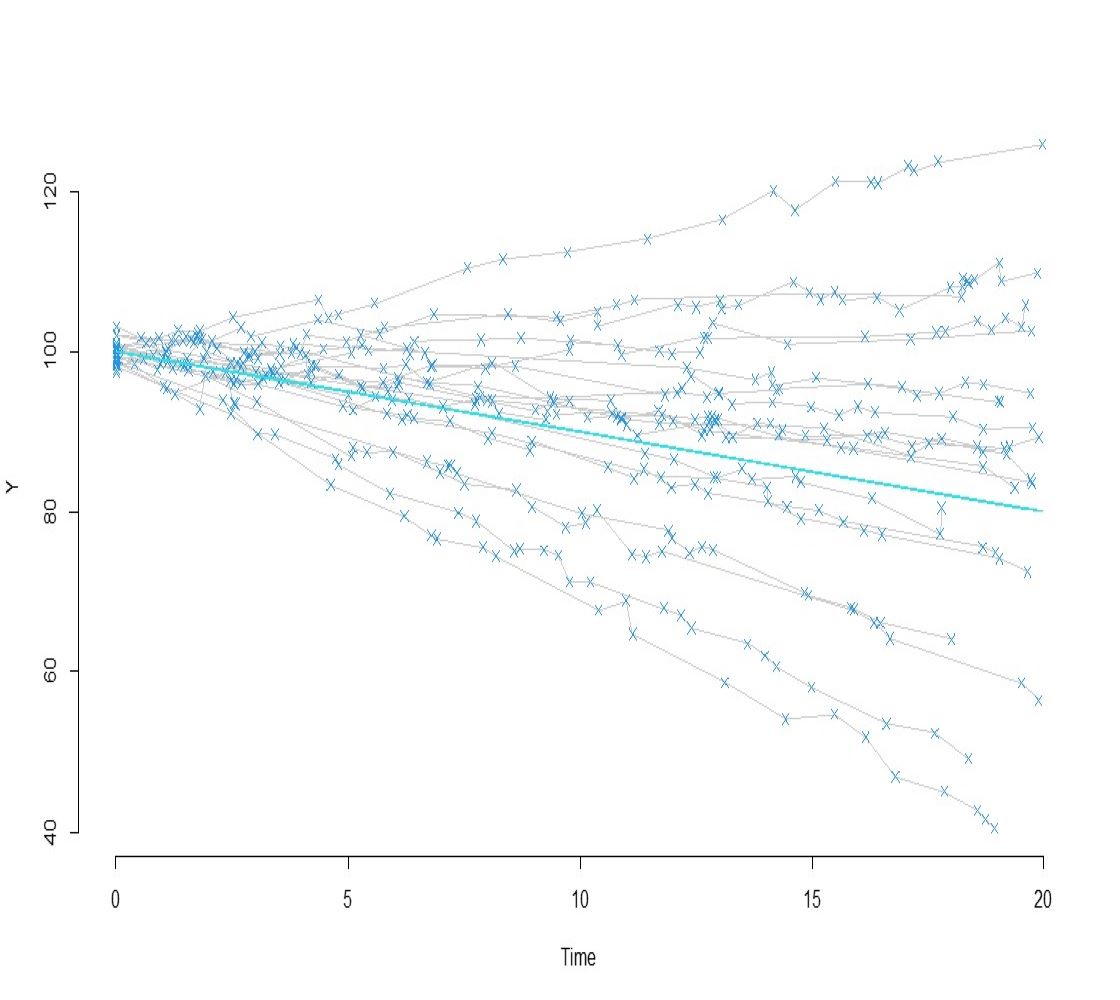
Health utilities
Health utilities are used in economic evaluations to help assess cost-effectiveness of treatments, and so ultimately contribute to decisions on which treatments should be publicly funded. We have shown that health utilities are measured subject to substantial uncertainty that is not correctly quantified, proposed solutions, and are currently developing more accurate approaches to utility measurement.
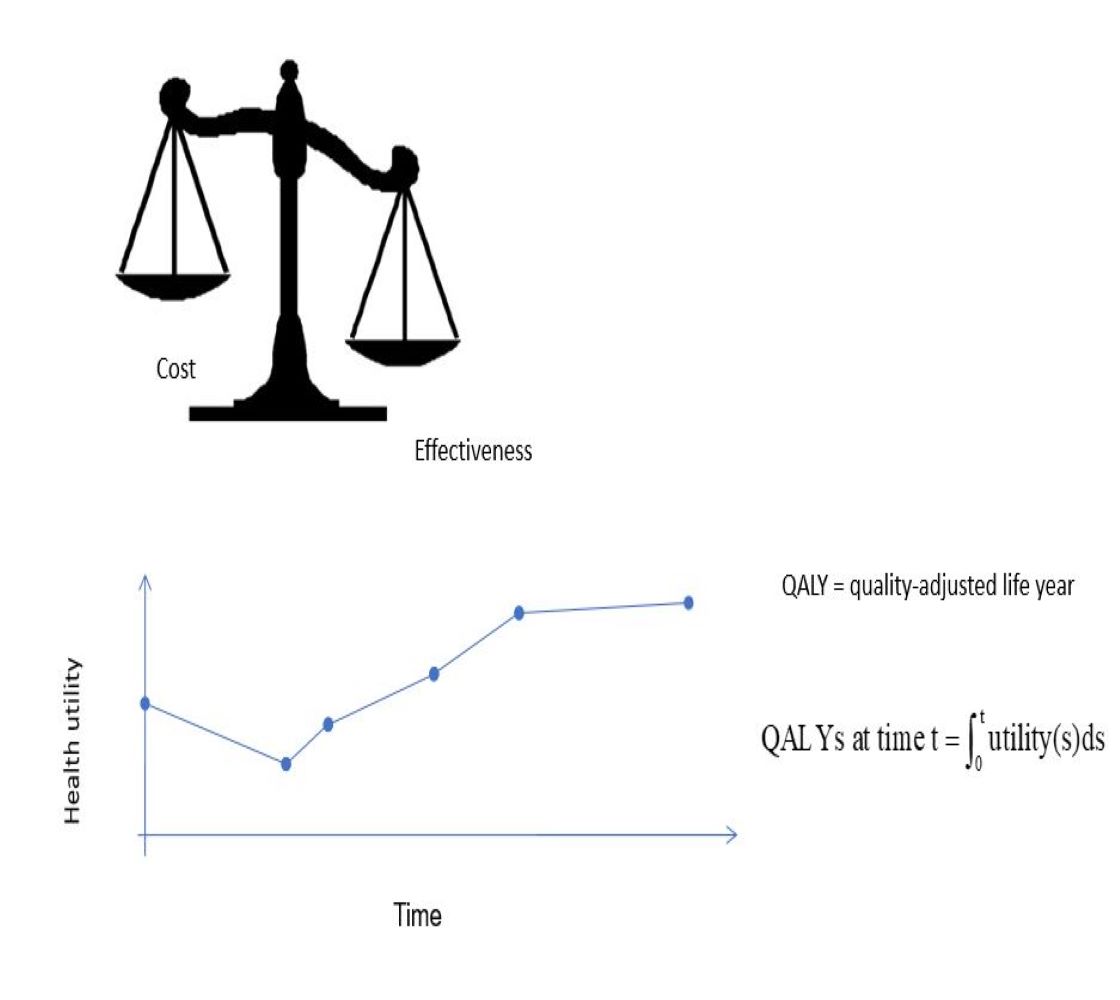
Knowledge translation in biostatistics
I am working on improving the uptake of new statistical methods in medical research. We began by listening to researcher’s opinions and preferences through an invited session at Statistical Society of Canada’s annual conference in 2014 and through a survey (Pullenayegum et al., 2016). This showed that collaboration and software were key facilitators in the uptake of new methods.
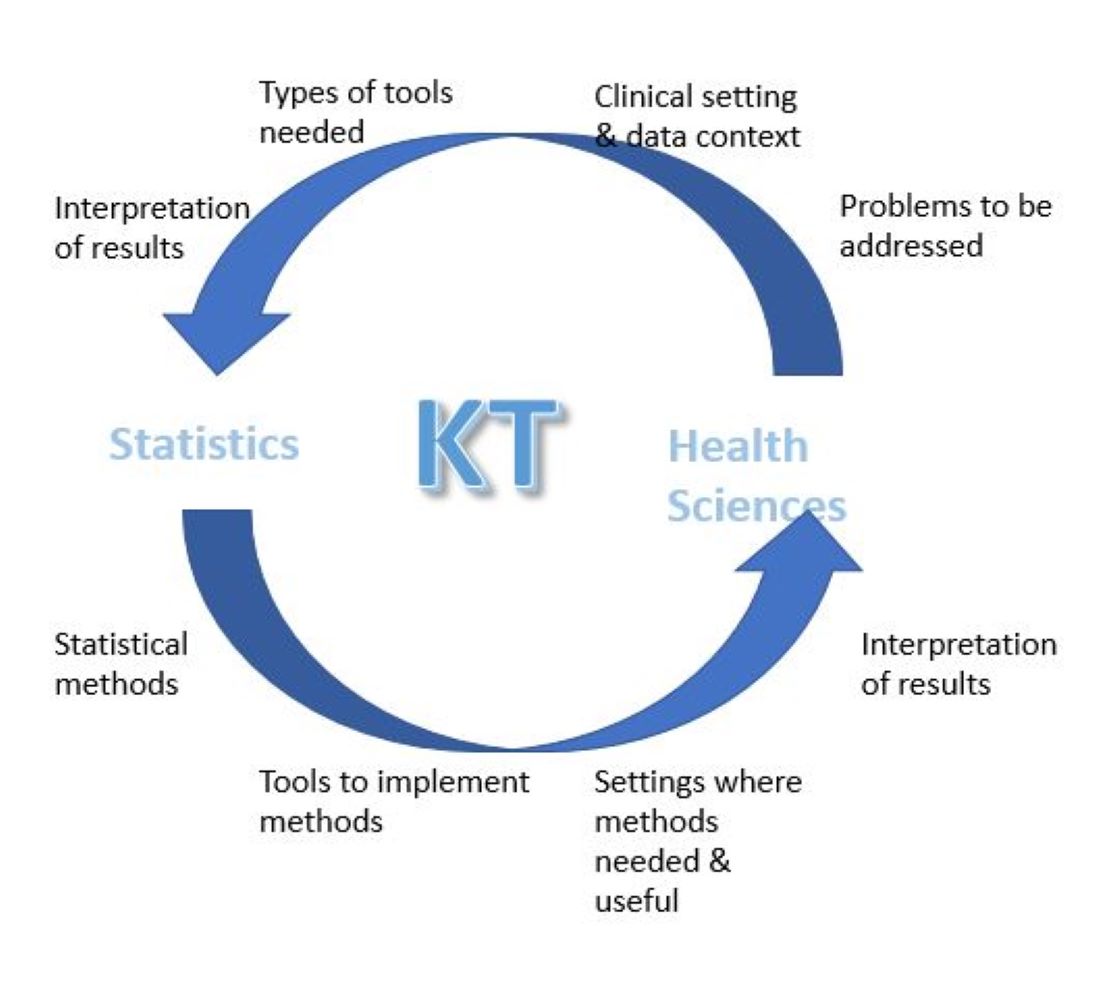
Individual Projects
Methodology for causal inference is rapidly expanding, however most methods have adopted a frequentist approach. We are investigating Bayesian approaches, via importance sampling and latent classes.
Longitudinal data is useful for describing disease trajectories. To date, focus has been on capturing population trajectories. This project is working on capturing subject specific trajectories through using an initial portion of the follow-up to predict long-term follow-up, and also selecting covariates (from a potentially high-dimensional set) to use in making predictions.
Valuation of the EuroQol instruments is currently underway. The recommended sample size of 1000 has proven infeasible for some studies, and so efficient approaches to analysis that offer the same predictive precision but with a smaller sample size would be helpful. Three non-parametric approaches have been proposed: one frequentist approach two Bayesian. These have led to 20-65% reductions in mean squared error. Sample size calculations using the US EQ-5D-3L suggest that the sample size could have been reduced three-fold when coupled with the non-parametric approach while still achieving better predictive accuracy than the full sample coupled with the traditional analysis.
These three approaches have never been compared to one another on the same dataset. Moreover, evidence that they lead to such substantial improvements in predictive accuracy in a range of datasets would provide more compelling evidence for their widespread use. The purpose of this project is to use EQ-5D-5L data from 9 countries to assess the reductions in sample size that could be achieved by using these alternative modelling strategies, and identify whether one method generally outperforms the others.
Most approaches to longitudinal data subject to irregular observation have been semi-parametric. Joint modeling of the longitudinal outcomes and gap times has been considered, but the models are difficult to specify correctly. However, recent work has suggested that parametric models are more robust to mis-specification than semi-parametric models. Patient charts often contain information about the recommended time to the next visit, and we will build on our previous work to incorporate this into the joint models.